Researchers at the Universities of Wisconsin and California are integrating physics and Machine Learning to predict materials degradation in nuclear reactors.
The reactor pressure vessel (RPV) is an essential component of the modern light-water reactor (LWR). The RPV encloses the reactor core, with a primary function of pressurising the coolant-moderator for more efficient operation, and a secondary function of providing a barrier to the release of potentially radioactive materials under normal or accident conditions. The RPV is made of steel and is very large (Fig. 1), typically approximately cylinder shaped with a diameter and height of around 5 metres and 10 metres, respectively, weighing hundreds of tons. The RPV can embrittle with time as a result of continued exposure to radiation, and this embrittlement can potentially become a safety concern. The RPV size and critical role in safety mean that it cannot be readily replaced or repaired. Therefore, understanding and predicting RPV materials properties is essential for planning the future of practical, safe operation of LWRs, such as extending licensing of existing plants to longer lifetimes (referred to here as ‘life-extension’).
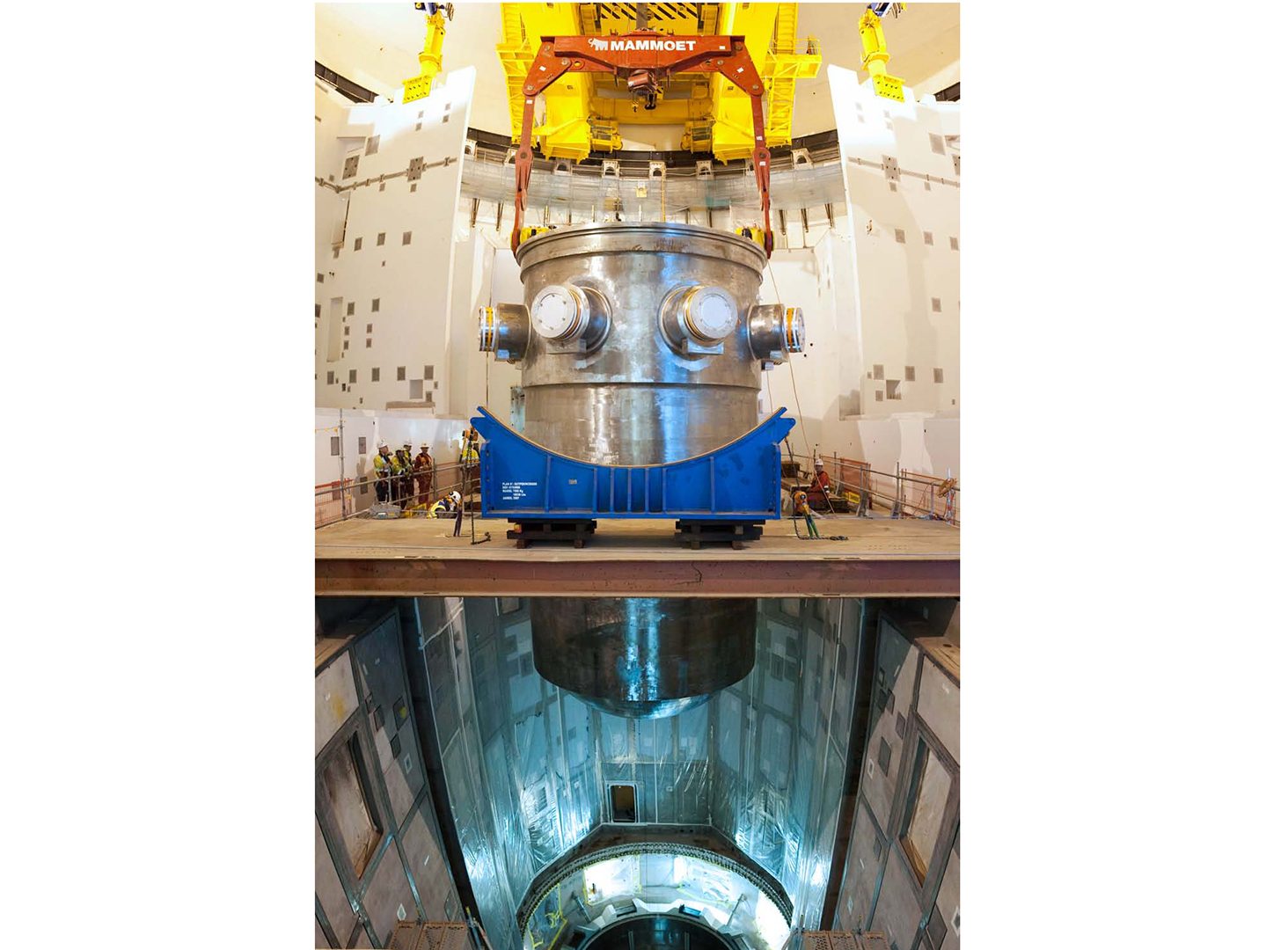
The importance of temperature shift
A particularly important property for a reactor pressure vessel is its ductile to brittle transition temperature, denoted Ts. Ts is the temperature below which the RPV becomes brittle and can easily be damaged by mechanical deformation. RPVs should be operated at temperatures above Ts. Ts is typically very low (around -50°C) for the steel in a new RPV, but the value of Ts shifts upward over time due to effects of neutron radiation from the reactor. This shift is primarily due to the formation of small precipitates in the RPV steel, making it harder to deform. If the ductile to brittle temperature shift, denoted ∆Ts, becomes too large, RPVs will be at risk of undergoing mechanical deformations below Ts and becoming damaged. ∆Ts is closely related to hardening of the material, typically quantified by the upward shift in yield stress, denoted ∆s, and both are used to quantify the embrittlement of the reactor pressure vessel. Predicting ∆Ts (and ∆σs) in RPVs is therefore an essential problem for assuring their safe use in LWR reactors in the future. Predictions are particularly important for pressurized water reactors (PWRs), where higher fluence leads to potentially concerning temperature shifts.
∆Ts and ∆σs can be readily measured, and excellent databases of thousands of values are available for different RPV and model alloy compositions, both from samples taken from actual reactors (surveillance data) and from samples irradiated in test reactors (test reactor data). Both these types of samples show that the values of the shifts depend sensitively on alloy composition (e.g., the amount of Cu, Mn, Ni, and Si alloying elements in the Fe-based steel) and environmental conditions. The most important environmental conditions are temperature, flux (number of neutrons per second per unit area hitting the metal), and fluence (total number of neutrons per unit area that have hit the metal). These databases provide excellent guidance on the behaviour of ∆Ts and ∆σs but have some significant limitations. In particular, surveillance data is constrained to flux and fluence values that have already occurred in operating reactors, and behaviour at higher fluence associated with life extension may be difficult to predict. Test reactor data can reach high fluence, but to make practical use of test reactors, any high fluence irradiations are necessarily done at flux values much higher than occur in LWRs. Therefore, shifts for low-flux, high-fluence life extension conditions cannot be directly measured, and extrapolation from existing databases is uncertain and is a focus of ongoing research. A map of the data available from surveillance reactors, as collected in the PLOTTER database, as well as from the large test reactor databases developed by Professor Odette at UCSB, is shown in Fig. 2 below. The region labelled 60-100 year operation are the highest fluence values likely to be seen in present LWR life-extension scenarios, and represent a flux and fluence where almost no data is available and reliable extrapolation approaches must be developed.
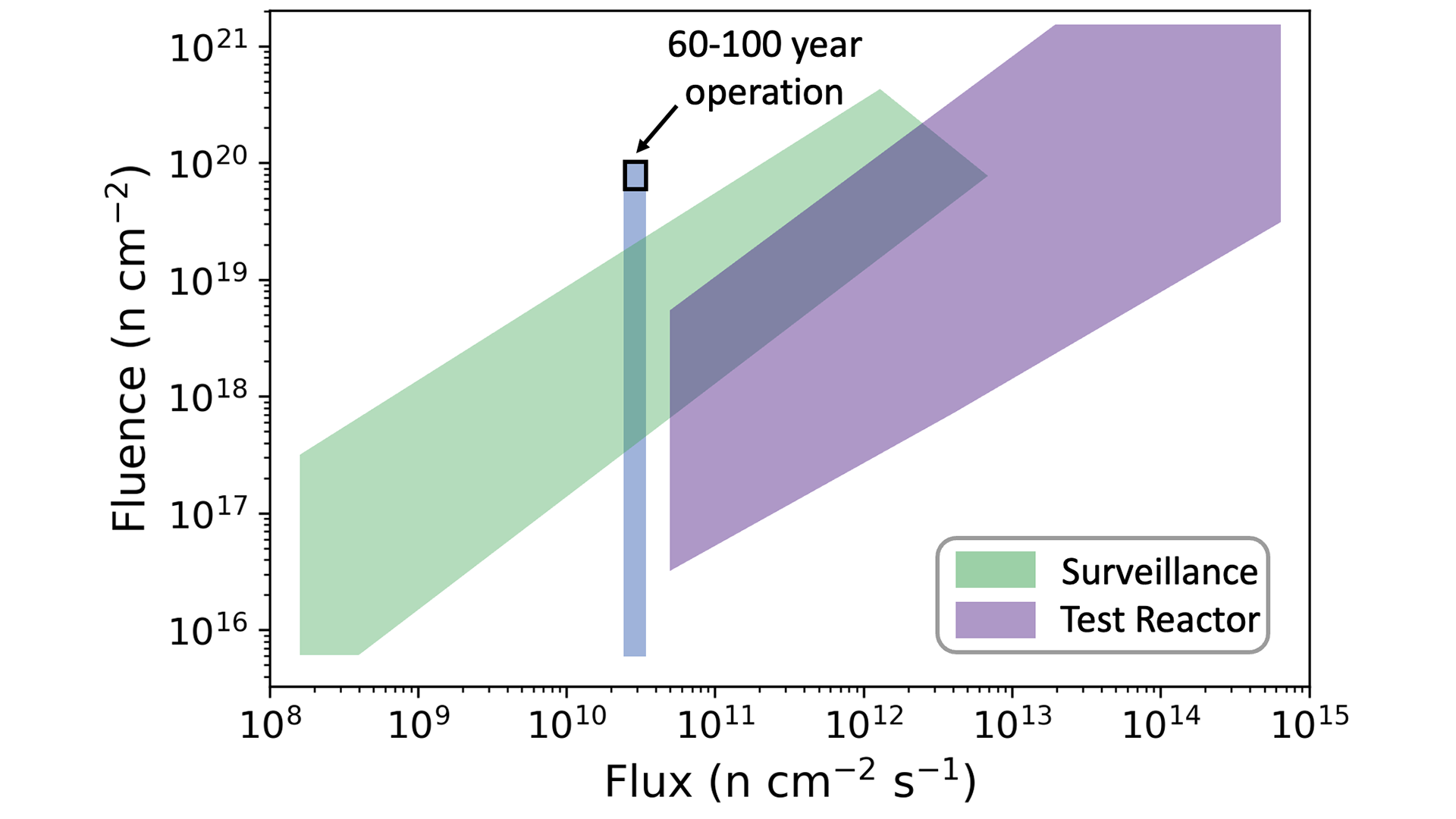
Modelling for RPV behaviour
There are three classes of models for reactor pressure vessel ∆Ts and ∆σs behaviour. The first class of models is what we will call ‘mechanistic’, which seeks to understand all the relevant materials transformations leading to the ∆Ts and ∆σs shifts. These models treat all (or at least most) the complex physics of radiation-induced changes in these materials, which includes everything from neutrons damaging the atomic scale crystal structure over a few trillionths of a second to cracks propagating through centimetres of material that has been aged 100 years. Such models have been the focus of major efforts in the US and Europe for decades and have yielded deep understanding of the mechanisms controlling ∆Ts and ∆σs, as well as some predictive ability. However, the complexity of the embrittlement process means that such models are still only semi-quantitative and cannot in themselves be relied on for robust predictions.
The second class of models are known as ‘physics-informed’. These models use varying amounts of mechanistic understanding and human intuition to develop functional forms for fitting, and then fit to existing databases. Well-known models used in multiple countries include Reg. Guide 1.99 Rev 2, JEAC 4201-2007 (2013 addenda), EONY, ASTM E900-2, and OWAY. These models are generally more accurate than fully mechanistic models for conditions close to the data used in their fitting, and for such conditions yield highly quantitative predictions (e.g., errors in ∆Ts and ∆σs are often just about 10°C and 15 MPa, respectively). However, since physics-informed models must make significant approximations to derive a practical functional form, and have to use functional forms with significant constraints, they can potentially miss key trends or overfit to their training data, so their ability to extrapolate to life-extension conditions is potentially uncertain.
The third class of models are what we will call ‘Machine Learning’ (ML), as they make use of the modern data-centric approaches of Machine Learning to train on the existing data and make predictions. These models generally have very little physics guiding them but utilise extremely flexible functional forms that can capture all of the complex data and can be developed rapidly. Machine Learning models have been explored for RPV shift predictions for over 15 years and are becoming increasingly popular due to improved understanding and tools associated with Machine Learning. These models can achieve errors matching the physics-informed models, but can be developed much more rapidly. However, their lack of underlying physics and their enormously flexible functional forms make them very susceptible to overfitting, and their ability to extrapolate to life-extension conditions is highly unclear. While each modelling approach has its strengths and weaknesses, all still have challenges yielding robust and trustworthy predictions when extrapolated.
Recently, a joint team from University of California – Santa Barbara, University of Wisconsin – Madison, and Oak Ridge National Laboratory have been funded by the U.S. Department of Energy (award number DE-NE0009143) Nuclear Engineering University Program (NEUP 21-24382) to help integrate existing databases and the above modelling approaches to yield more robust and quantitative predictions of ∆Ts and ∆σs. This group has decades of experience in reactor pressure vessel modelling, including mechanistic, physics-informed, and Machine Learning approaches, and now seeks to integrate them for greater accuracy. The team is generating an unprecedented training database by combining the most recent surveillance database and test reactor databases which is challenging due to some databases, including only ∆Ts and some only ∆σs, respectively.
The team is then seeking to integrate best practices from all of the above modelling approaches, developing Machine Learning models that are highly accurate, physically informed, and validated against existing understanding of RPV embrittlement mechanisms. Such approaches may yield highly accurate models that can be trusted to extrapolate to life extension conditions, and potentially to new alloys to aid in the development of new reactor pressure vessel materials. More generally, the successful integration of physical and Machine Learning approaches for predicting radiation effects in RPVs can form a foundation for modelling radiation effects across many materials, supporting not just our present reactor fleet but next-generation technologies like fast or traveling wave reactors.