Researchers from the MRC Brain Network Dynamics Unit and Oxford University’s Department of Computer Science have shown how the brain learns compared to Artificial Intelligence systems.
A team of researchers have studied how the brain learns – identifying the fundamental principle employed by the brain during learning.
They looked at existing mathematical equations describing changes in the behaviour of the brain’s neurons, and the synaptic connections between them.
The team then analysed mathematical models and found that the brain employs a different learning principle to those used by Artificial Intelligence systems.
The research, published in Nature Neuroscience, may now inspire faster and more robust learning algorithms in AI.
How is the brain superior to AI systems?
The biological brain is superior to current Machine Learning systems, as we can learn new information by just seeing it once. Artificial systems, however, need to be trained hundreds of times with the same pieces of information to learn them.
We can also learn new information whilst maintaining the knowledge we already have. In artificial neural networks, on the other hand, learning new information can interfere with existing knowledge, degrading it rapidly.
How the brain learns versus AI system learning
The researchers believe that the human brain first settles the activity of neurons into an optimal balanced configuration. Then, synaptic connections are adjusted. The way the brain learns is extremely efficient, reducing interference by preserving existing knowledge.
In artificial neural networks, an external algorithm attempts to modify synaptic connections to try and reduce error.
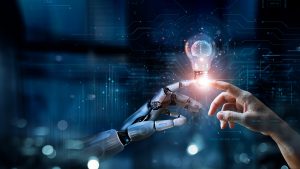
The team argue that AI systems that employ the learning method of the brain can learn faster and more effectively than other artificial neural networks in tasks that are typically faced by animals or humans in nature.
Example to demonstrate the difference in learning methods
The team illustrate the difference between how the brain learns compared to an AI system with the example of a bear fishing for salmon.
The bear can see the river, and has learnt that it is likely to catch a salmon if it can hear the river and smell the salmon. One day, however, the bear arrives at the river with a damaged ear, so cannot hear it.
In an AI processing system, the lack of hearing would result in a lack of smell. This is because when the system learns there is no sound, backpropagation would change some of the connections, including those between neurons encoding the river and the salmon. In this instance, the bear would conclude that there is no salmon and would go hungry.
However, in an animal’s brain, the lack of sound does not interfere with the knowledge that there is still the smell of the salmon, and the salmon is likely to be in the river for catching.
A lack of interference during learning
The researchers developed a mathematical theory to show how the brain learns – due to a lack of interference between information during learning. In the brain, the neurons settle into a prospective configuration that reduces this interference.
The team showed that prospective configuration explains neural activity and behaviour in a number of learning experiments, better than artificial neural networks.
Lead researcher Professor Rafal Bogacz of MRC Brain Network Dynamics Unit and Oxford’s Nuffield Department of Clinical Neurosciences said: “There is currently a big gap between abstract models performing prospective configuration, and our detailed knowledge of anatomy of brain networks.
“Future research by our group aims to bridge the gap between abstract models and real brains, and understand how the algorithm of prospective configuration is implemented in anatomically identified cortical networks.”
The first author of the study, Dr Yuhang Song, added: “In the case of Machine Learning, the simulation of prospective configuration on existing computers is slow because they operate in fundamentally different ways from the biological brain.
“A new type of computer or dedicated brain-inspired hardware needs to be developed, that will be able to implement prospective configuration rapidly and with little energy use.”