Modern techniques in probability theory and machine learning are contributing to making fusion energy a reality.
Fusion devices are extremely complex machines, with many physics and technology challenges to be overcome on the road to fusion energy development.
The infusion research unit at Ghent University (UGent) specialises in the field of data science, with applications to a wide variety of data-related problems in fusion devices based on magnetic plasma confinement. This is the line of fusion research wherein strong magnetic fields are used to confine the extremely hot gas, or plasma, in a doughnut-shaped configuration known as a torus.
Such devices, called tokamaks and stellarators, presently constitute the most advanced type of fusion machines, and they will most likely lead to the first electricity-producing fusion power plants. That is if fusion scientists succeed in overcoming a number of scientific and technological hurdles that presently stand between the existing laboratory experiments and a pilot plant.
Fusion data science
To make this possible, data from experiments and computer simulations need to be processed and analysed – lots of complex data.
In order to ensure the safe and efficient operation of a fusion device, a multitude of sensors capture key information about the plasma and a wide range of machine subsystems. These sensors provide signals of quantities like temperature, density, magnetic field, etc.
More recently, images and video data have been increasingly produced. This leads to massive volumes of data that need to be processed or analysed in detail. For instance, the ITER fusion experiment is estimated to produce up to two petabytes (two quadrillion bytes) of data per day.1 Furthermore, some measurements need to be available routinely and processed with minimal delay, notably for feedback control of the plasma state.
In addition to data volume and requirements on the processing speed, all measurements are affected by uncertainty to varying degrees. In other words, the raw measurement taken by a sensor (usually a voltage) gives an estimate of the properties of the plasma or machine components, but inevitably this comes with some measurement error. Scientists often rely on physical models to describe plasma behaviour, but this also introduces uncertainty since no model is perfect.
As a result, physicists and engineers aiming to better understand or control fusion plasmas and fusion devices are faced with a daunting challenge when trying to interpret their data. This is where the work of the infusion group at UGent comes in. We have been at the forefront of this research for more than 20 years.
Perhaps surprisingly, the application of dedicated, advanced methods for analysing the data is a relatively recent evolution in fusion R&D. Whereas basic statistical methods have been used for estimating trends in large, multi-machine databases, more modern analysis techniques have seen a wider acceptance in the field only since about 10-20 years.
It is also important to note that data science, in the sense used here, is an interdisciplinary field involving classical statistics, probability theory, machine learning, artificial intelligence (AI), as well as methods for data management, basic data processing (e.g. data cleaning), visualisation, etc. So, not every data scientist is a statistician, while machine learning and AI only cover certain aspects of data science, each with its own strengths and application areas.
At UGent, we work in a number of areas in collaboration with several other universities and fusion laboratories around the world:
- Discovering meaningful patterns in complex data sets.
- Probabilistic modelling of seemingly random plasma properties, like turbulent fluctuations and sudden plasma events.
- Joint processing of data from multiple sensors in order to extract a maximum of information from a minimal amount of data.
- Detection and prediction of unexpected events and failures of machine components for optimising maintenance strategies.
Let us look at a few examples to illustrate these activities.
Pattern recognition for fusion data
In complex systems like a fusion device, important properties that ultimately determine the power output of the machine depend on many other parameters that can be controlled, at least to some extent, by the designers and operators of the machine.
For instance, it is well known that the ability of the magnetic field to confine the heat of the plasma improves with the size of the device. This is intuitively clear: it simply takes more time for the heat to escape from a larger device. This is one of the main reasons why fusion machines using magnetic confinement are so large.
But how exactly does the heat confinement depend on machine size? Does it scale proportionally, or is there a more complicated relation? Answering this question is directly relevant to the design of new machines. Moreover, there are many other machine parameters that determine the performance in terms of fusion power – more knobs to turn in order to obtain the best conditions.
One way to characterise these various dependencies relies on the analysis of large databases consisting of measurements obtained from many experiments at various fusion devices. Then, using specialised statistical methods, it is possible to capture the trend of confinement (the thermal energy confinement time τE, th, to be precise) in terms of machine size and other relevant parameters.
This is illustrated in Fig. 1, showing the approximately linear trend of confinement with the major radius Rgeo of the devices. This result was obtained using a recent update of one of the main fusion databases, which was compiled through a major international collaboration.2
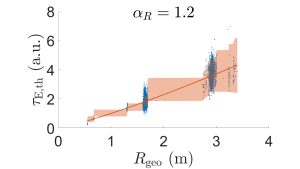
(major radius Rgeo) in high-confinement tokamak plasmas
Moreover, an important asset of probabilistic methods is that they allow quantifying the uncertainty of estimates or trends, as depicted in the figure by the red-shaded confidence band.
Fluctuating plasma events
Another application where probability plays a major role is in characterising fluctuating plasma phenomena. These are physical events that can be extremely difficult to describe or control, exhibiting irregular, random behaviour.
Such events are quite common in nature, ranging from earthquakes to solar flares or complex flow patterns in rivers and oceans. In fusion plasmas, the flow of energy and particles from the hot plasma core to the wall also occurs in an irregular, turbulent way.
On a microscopic scale, this causes local fluctuations of plasma properties like density and temperature. Other events exhibiting randomness occur on much larger scales, like certain plasma instabilities. Some of these can cause significant outbursts of heat and particles that may pose a significant threat to the wall materials.
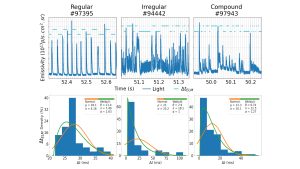
Fig. 2 shows the peaks of light emission from the plasma edge in the JET tokamak (Culham Centre for Fusion Energy, UK) caused by a type of plasma instability called an edge-localized mode (ELM).3,4 The characteristics of the ELMs, like the time between two ELMs and ELM size, can vary significantly from one burst to another but also between different experiments (plasma discharges).
In the three plasma discharges shown, the time ΔtELM from one ELM to the next may be very difficult to predict. Yet, the probability distribution (lower panels) exhibits a unique structure that can be captured by probability models like the Gaussian or Weibull distributions. In turn, this approach offers a better understanding of the underlying physical mechanisms, ultimately allowing us to mitigate or entirely avoid these large, dangerous plasma outbursts.
Sensor fusion
One of the main activities of the group lies in the area of sensor fusion. Whereas the fusion of atomic nuclei provides the origin of fusion energy, sensor fusion refers to the joint processing of data captured by multiple sensors in order to maximise the information obtained from the experiments.
This is certainly no luxury, considering the significant measurement difficulties in the harsh plasma environment, as well as the limited space that will be available for sensors in future reactors. We use a probabilistic framework known as Bayesian inference, after the scientist Reverend Thomas Bayes, who contributed to the foundations of the field in the 18th century.
Fig. 3 shows a cross-section of one possible design of a generation of future demonstration fusion power plants (DEMO), along with the locations (coloured dots) of the sensors that measure the magnetic field used to confine the plasma.5
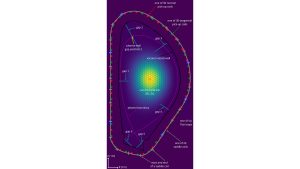
By merging the data from all sensors using the Bayesian framework, an accurate reconstruction of the position of the plasma inside the torus becomes possible. This is essential to prevent the hot plasma from touching the walls of the device, keeping it safely confined inside its magnetic cage. We have applied similar tools to measure the concentration of tungsten particles originating from the wall due to the constant exposure to plasma leaking out of the cage.
Fig. 4 shows an example of a build-up of tungsten particles in the core plasma of the WEST tokamak, based on measurements of X-rays emitted by the tungsten impurities.⁶ Again, the probabilistic approach allows uncertainty estimates, visualised by the error map in the right panel.
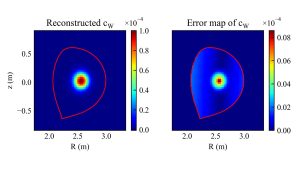
Towards a fusion power plant: anomaly detection and predictive maintenance
Until recently, fusion research has been mainly the work of scientists in large-scale, publicly funded laboratories. However, as the science behind fusion has steadily matured, the focus of the activities has shifted gradually to the technological aspects. Hence, the role of engineers and the supporting industry has become increasingly important, to the point that today, there is significant private investment in fusion R&D. With public-private partnerships on the rise, joint efforts involving public and private sectors are boosting the development of fusion energy. In the process, there is an important analogy to be made with the rise of commercial aviation in the second half of the 20th century. At the time, data gathered from wind tunnel experiments contributed to a booming aerospace industry. In a similar vein, data-driven fusion research has the potential to accelerate the advent of fusion energy.
One of the areas where data-centric discovery can help address technological challenges is anomaly detection and predictive maintenance.
By using sensors to regularly or continuously monitor the condition of equipment or products in an experimental setting or in a production environment, it is possible to train a computer model to recognise abnormal events in the operation or the state of the monitored system. This can make a crucial difference in protecting hardware or for quality assurance, either by raising an alarm to allow human intervention or by automatically activating counter-measures to restore the system to its nominal state.
Predictive maintenance is a strategy that takes this one step further: using statistics or machine learning techniques, a computer can be trained to recognise early signs of an upcoming anomaly or failure. Machine learning models like neural networks are especially well suited to pick up subtle warning signs of an imminent failure while still allowing sufficient time to take appropriate action.
The complex environment of a fusion device can benefit greatly from such new techniques that are also quickly gaining popularity in many sectors of industry.
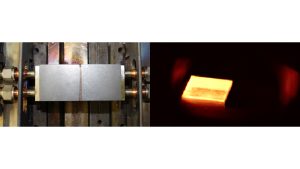
Some concrete examples that we have worked on are the operation of pumps ensuring the vacuum in fusion devices and large circuit breakers that are essential for plasma start-up in tokamaks. A recent use case, illustrated in Fig. 5, involves the monitoring of wall components with infrared cameras in order to detect or predict material overheating due to plasma exposure.7
Fusion education at UGent
Finally, as a research unit at a university, the infusion group is also active in university education. With fusion R&D booming, there is a strong need for highly skilled scientists and engineers to bring fusion electricity to the grid as soon as possible.
Because fusion is a uniquely interdisciplinary yet specialised domain, not one institution has all the necessary in-house expertise to effectively train students in a comprehensive way. This is why our group has been involved in fusion education at an international level for almost 20 years.
The European Master of Science in Nuclear Fusion and Engineering Physics (FUSION-EP) is an EU-funded collaboration between eight higher-education institutions in five European countries.8,9 It offers a two-year master’s programme around the physics of fusion plasmas and the technology of fusion devices. Students from all over the world enter this programme to benefit from a unique blend of expertise offered by the core partner universities and a selection of top fusion laboratories, including the ITER Organization and FuseNet, the European Network for Fusion Education.
The programme includes several weeks of experimentation at fusion labs, as well as the opportunity to conduct cutting-edge research at one of the partner universities or labs, leading to the master thesis.
In addition, we are involved in a joint PhD programme with Czech Technical University in Prague, in close collaboration with several renowned fusion labs, notably the Institute of Plasma Physics of the Czech Academy of Sciences.
At infusion, we are proud to be part of an endeavour that combines two of the greatest challenges of our time: sustainable energy supply and data science. Through original research supported by strong mathematical foundations but with a keen eye for concrete impact, we are contributing to making fusion energy a reality. Combined with our efforts in training the next generation of fusion scientists and engineers, we are confident that we are making a tangible contribution to developing fusion as a clean, safe and sustainable source of energy.
References
- https://www.iter.org/node/20687/how-manage-2-petabytes-new-data-every-day
- G. Verdoolaege et al., Nucl. Fusion 61, 076006, 2021
- G. Verdoolaege et al., Proc. 45th EPS Conference on Plasma Physics, P2.1078, Prague, 2018
- J. Alhage, G. Verdoolaege et al., 5th IAEA Technical Meeting on Fusion Data Processing, Validation and Analysis, Ghent, 2023
- J. De Rycke, G. Verdoolaege et al., 21st International Congress on Plasma Physics, Ghent, 2024
- H. Wu et al., 21st International Congress on Plasma Physics, Ghent, 2024
- L. Caputo et al., 21st International Congress on Plasma Physics, Ghent, 2024
- G. Van Oost et al., Eur. J. Phys. 42, 024002, 2021
- https://fusion-ep.eu
Please note, this article will also appear in the 20th edition of our quarterly publication.