Nuno Mendes, Head of Product Management at Stratio, highlights how AI predictive maintenance can enhance the socio-economic and environmental impact of public transport.
The high costs of owning and maintaining a car in today’s financial climate, coupled with a declining public transport system, are contributing to transport poverty across the UK. Studies have shown that ensuring access to good transport systems makes it three times more likely that people can access vital services such as education and healthcare, or employment opportunities. However, despite the increasing financial burden and environmental concerns associated with car ownership, private vehicles remain the preferred mode of transportation for most commuters. This means that cars continue to represent a significant contribution to greenhouse gas emissions across Europe, and congestion is a persistent urban problem. Reforming our approach to shared mobility is therefore a crucial strategy to address socio-economic and environmental concerns alike.
Of course, in order to find successful solutions to a problem we have to first understand what is causing it. So, what is at the root of consumers’ reluctance to choose the bus over the car, despite the obvious drawbacks of the latter? Service reliability is the answer. Vehicle breakdowns, resulting in service disruptions and downtime, erode passengers’ confidence in bus routes and networks, discouraging them from using public transport. This leads to revenue loss for operators, increased fares, and service reductions. It’s a vicious cycle of transport decline.
The key to increasing bus usage, therefore, lies in enhancing the reliability of bus networks and re-building consumer confidence; ensuring that buses remain on the road and in service, getting commuters where they need to be, when they need to be there. This focuses our attention on the means to reliability: effective maintenance strategies that work towards the goal of zero downtime for buses.
Choosing the right maintenance strategy
Emergency repairs are often expensive, involving recalling a vehicle and paying for short-notice delivery of parts. This is particularly true for electric buses which have far higher associated component and labour costs. Combined with the lost revenue from reduced passenger numbers, this can have a negative impact on the bottom line. So, it is not surprising that fleet operators have already been working to avoid emergency repairs and extend the life cycle of vehicle components to reduce downtime, bring down increasing maintenance costs, improve reliability, and protect revenue streams. However, with both reactive and preventive strategies experimented with, neither have achieved the ideal of zero downtime, failure prevention, and cost reduction.
Reactive maintenance for instance, although it saves money in the short term, involves repairing a vehicle after a problem has already occurred. This means expensive emergency repairs requiring short notice delivery of parts, and potentially the rental of a replacement bus, which can be even more costly. Additionally, but equally important, recalling the bus from the road and disrupting service routes negatively affects the customer experience.
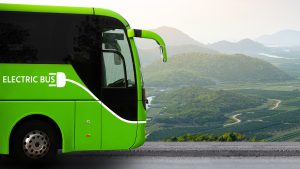
On the other hand, while preventive maintenance can prolong a vehicle’s lifespan by completing regular checks based on estimations of when a failure might happen, the approach does not optimise costs. In fact, it often leads to more time spent servicing a vehicle that may not require it, thereby keeping buses off the road and once again impacting customer service.
Using AI for transport analytics
By leveraging Artificial Intelligence (AI) and Machine Learning to automatically collect and analyse vehicle data, predictive, AI-enabled maintenance represents the most advanced method for achieving reliability in transportation. Providing fleet managers with real-time insights into individual bus components, it allows for more accurate scheduling of maintenance and repairs, improving fleet utilisation, and reducing costs. Additionally, vehicle breakdowns can be prevented by predicting equipment failure, reducing downtime, and protecting revenue and customer satisfaction.
Compared to preventive maintenance, which requires visual inspections and assumes all vehicles have the same requirements, predictive maintenance accounts for variations between vehicles assigned to different routes. For instance, a city bus will brake more frequently than a long-haul vehicle, and AI predictive maintenance can identify patterns in the data to predict when, for example, a brake pad replacement will be necessary. This allows for bulk ordering of parts and scheduling of maintenance during off-peak periods, reducing the costs associated with unplanned or pre-emptive part replacement and minimising downtime.
AI-enabled predictive maintenance solutions, by virtue of requiring a complete vehicle data collection, also enable the digitisation of repetitive tasks, such as odometer readings, coolant checks, and oil changes, ensuring a higher degree of accuracy over manual checks and freeing up teams to perform higher-value tasks. Furthermore, the system proactively alerts engineers of possible risks, enabling improved service planning.
Facilitating the transition to electric buses
The insights enabled by a predictive maintenance approach are particularly crucial for operators transitioning to electric vehicles (EVs), which are generally more expensive than traditional internal combustion engine (ICE) buses. By using predictive maintenance and predictive battery analytics, operators can keep their EVs on the road for a longer duration and distance, achieving cost neutrality more quickly. The extended life of the battery packs also benefits the return on investment for the purchase of electric buses since the cost can be spread over a more extended period.
As with ICE vehicles, a preventive approach to maintaining EVs is ineffective from a service and cost perspective. The far higher component and labour costs make the replacement of components before they have reached end of life in order to prevent breakdowns and avoid service disruption highly inefficient for operators deploying EVs.
However, by integrating predictive solutions that collect and analyse vehicle data during regular operation, fleet managers are able to establish the true remaining useful life (RUL) of components and use the information to intelligently schedule vehicle servicing in order to extend operational life. Moreover, by gaining real-time, actionable insight into the internal faults of electric buses, maintenance managers can diagnose malfunctions remotely. Vehicles stay on the road for longer, maintenance becomes more predictable, and less expensive and breakdowns happen less frequently, preventing costly downtime and service disruption.
EV batteries represent the most stark example of the benefits of increasing the lifespan of components. Accounting for around 40% of the total vehicle cost, public transport managers need to take action to extend the battery’s life cycle if they hope to achieve a profitable shift to electric fleets. Calculations must be made to account for the degradation of the battery over time and the impact this has on range, as well as a range of factors beyond their control such as weather, traffic, route, and load which can alter the distance a bus can travel on one charge.
Without this understanding and visibility, it will be impossible to plan for a smooth, efficient and cost-effective service. But predictive battery analytics can provide an accurate, comprehensive view of the battery health evolution of an EV bus, allowing for effective route planning and charging requirements, as well as usage optimisation metrics to extend the lifespan of the vehicles. By leveraging how the battery packs are being used through State of Charge (SoC) and Depth of Discharge (DoD) data, fleet managers can understand if the operation profile can be changed to maximise battery life, reducing the total cost of ownership of electric buses. This type of analysis is fundamental for an operationally successful and profitable EV fleet deployment.
Achieving the lowest cost per mile financially and environmentally
With deadlines for the elimination of ICE vehicles on UK roads fast approaching, the transition to EV vehicles is more a question of when than if for public transport providers – and will obviously significantly impact the environmental footprint of public transport. However, in order to also make a difference in social and economic terms for those experiencing transport poverty, and to further encourage private car users back onto public transport, operators will also need to combine the sustainability of EVs with reliability, accessibility, and profitability – achieving the lowest cost per mile. This can be achieved by extending the life of components, operating vehicles more intensively and for much longer hours, while keeping them out of the workshop and on the road by eliminating downtime.
If this can be done, then bus services can reverse the decline of recent years. Ensuring service reliability and getting people back onto public transport will increase operators’ revenue and reduce transport poverty, connect people with opportunities and services, reduce car reliance, and alleviate environmental pressures. Thus, reliable public bus networks supported by AI-powered predictive maintenance will serve the ecological, social, and economic needs of future generations.
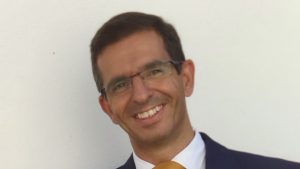
Nuno Mendes
Head of Product Management
Stratio