Experts from the Toyohashi University of Technology have innovated an Artificial Intelligence (AI) model that simultaneously handles the perception and control of an autonomous vehicle.
The AI model proficiently perceives the surrounding environment via various vision tasks while controlling the autonomous vehicle as it follows a series of route points. The model can safely navigate the vehicle through diverse environmental conditions under a range of scenarios and has been tested with point-to-point navigation tasks, achieving the best drivability among recently tested models in a standard simulation setting.
Challenges with autonomous driving
Autonomous driving is an extremely complex system comprising various subsystems that manage perception and control tasks. Nevertheless, using multiple task-specific modules is expensive and inefficient because multiple configurations are still required to form an integrated modular system.
Additionally, the integration process can result in lost information as many parameters are manually adjusted. However, this can be combatted with rapid, deep learning research to train a single AI model with end-to-end and multi-task manners.
This enables the model to provide navigational controls to the autonomous vehicle based on observations made by sensors. Manual configuration is no longer required as the model can manage the information itself.
The difficulty that remains for an end-to-end model is how to extract useful information for the controller to estimate the navigational controls properly. This can be overcome by providing substantial amounts of data to the perception module so that it can better understand the environment. Additionally, a sensor fusion technique can be implemented to elevate performance as it fuses different sensors to obtain multiple data aspects.
However, this larger model is needed to process more data and causes a substantial computation load. Furthermore, it requires a data preprocessing technique as multiple sensors can produce different data modalities. Imbalance learning during the training process can also cause issues as the model performs perception and control tasks at the same time.
Refining AI
To overcome these limitations, the researchers developed an AI model trained with end-to-end and multi-task manners that included two main modules – the perception and controller modules. The perception phase starts by processing RGB images and depth maps from a single RGBD camera.
Information is then extracted from the perception module in addition to vehicle speed measurement and route point coordinates which are decoded by the controller module to estimate the navigational controls.
To guarantee that all tasks are performed equally, the team utilise an algorithm called modified gradient normalisation (MGN) to balance the learning signal throughout the training process. The team used imitation learning, allowing the model to learn from a larger dataset to achieve a near-human performance. The researchers also designed the AI to use a smaller number of parameters than others to reduce the computational load.
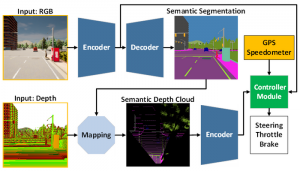
Using a standard autonomous driving simulator known as CARLA showed that fusing RGB images and depth maps to create a birds-eye-view (BEV) semantic map boosted overall performance. This is because the perception model better understands the scene, and the controller module can utilise data to estimate the navigational controls optimally.
The team concluded that the AI model is preferable for deployment in autonomous vehicles as it achieves better drivability with fewer parameters than other models. They are now working to improve the model to perform better in poor illumination conditions, such as at night and in heavy rain.