DIGI4ECO aims to boost new discoveries for the management and restoration of marine ecosystems by combining demo-mission data with historical and sleeping data for spatiotemporal modelling.
The rapid advancement of digital technologies, such as Digital Twins and artificial intelligence (AI), has opened up promising avenues for ecological actions, including conservation, research and restoration. These technologies offer improved monitoring capabilities that can enhance our understanding of ecological processes and biodiversity. In this framework, one of the fundamental European Digital Twin Ocean (DTO) missions aims at the digital representation of the physical ocean and its bio-ecological processes.
Many hurdles still exist in populating DTO virtual infrastructure with historically stored or newly acquired data. The challenges of feeding a DTO also include the harmonisation, real-time coverage, and data collection of wide areas, advanced prediction capabilities, and its correlation with human-nature-generated actions. Moreover, autonomously operating permanent systems for the monitoring of marine activities are multiplying worldwide, and an increase in the coordinated data collection capability would provide innovative multidisciplinary data for scientific, industry, and civil stakeholders.
The mission
The EU-funded DIGI4ECO project aims to develop a DTO-sustained 4D ecological monitoring system made by networks of robotic platforms such as Physical Twins of the Ocean (PTO), which is tailored for restoration efforts in fishery-depleted areas.
Hierarchic ecological indicators suited for monitoring network technologies (e.g. from local species counts and animal sizing to area species densities, biomass, and overall richness and evenness) will be computed, merging in-situ data from demo missions with locally available data from societal actors (e.g. fishery associations) and marine institutions.
Those indicators will provide a holistic 4D data collection in the water column and seafloor, expanding our knowledge capacity in data acquisition, storage, elaboration, and processing.
The project will use a cooperative group of autonomous stationary platforms as cabled observatories and landers (deployed by opportunity vessels) and mobile robots as Autonomous Underwater Vehicles (AUVs), Internet-operated Vehicles (IOVs) as crawlers, Unmanned Surface Vehicles (USVs), and pelagic arrays (i.e., Angler camera systems).
Some of these platforms will also be equipped with innovative sensors, such as omics eDNA sampling tools for laboratory sequencing.
The DTO architecture will offer a 4D holistic view of the monitored areas (i.e. demo sites) and their surroundings (i.e. geographical scaling). By merging real-time, SCIENTIFIC, and socioecological data, the DTO will enable oceanographic and socio-ecological modelling studies to assess the spatiotemporal dynamics of ecological indicators in demo-mission areas.
The DTO will be fed with processed data by AI algorithms that allow the identification and classification of species for the computation of ecological indicators in near real-time.
Data standards for harmonisation and quality control
Nowadays, there is a lack of standards for harmonising and guaranteeing the quality of data. Some institutions implement different tools to solve this gap (e.g. by using the Open Geospatial Consortium (OGC) resources applied to the metadata). Different methods for acquiring data exist, such as the PUCK protocol standard, SensorML, or Sensor Observation Service, all of which are from OGC.
Nonetheless, these tools and methods are best practices, and an effort to make them the gold standard and used throughout the community is still required. DIGI4ECO will implement new procedures to ensure data quality and harmonisation. This effort will facilitate data ingestion and integration into the DTO architecture.
Additionally, developing new tools and practices to harmonise the data will boost their applicability in different areas, such as AI classification (see next section), where the lack of standardisation makes it difficult to train the same CNN with images from different institutions/setups, a problem called ‘distribution shifts’. In support of that effort, this project will collaborate and search for synergies among open-source initiatives worldwide (e.g., FathomNet).
At the same time, DIGI4ECO will address the lack of tools to effectively extract, harmonise, and publish historically-stored data in numerous national and marine institutes, fishery associations, and research groups. This has been noted as one of the main barriers to increasing stakeholders’ and policymakers’ access to data. Therefore, one of the DIGI4ECO’s main objectives is to integrate the digital biodiversity component into the DTO architecture by consolidating already implemented data standards, near-real-time data quality control procedures, and communication protocols between data centres.
To pursue long-term uses of the DTO, commitments among data owners will be agreed to integrate more sources of biodiversity data. Furthermore, the project will develop protocols facilitating the automatic integration of data in the DTO and their assimilation into biodiversity/ecosystem models. This will allow the flow of data to continue and remain sustained after the end of the project, respecting the Findable, Accessible, Interoperable, and Reusable (FAIR) principles.
Enhancing data modelling
EU promotion of open science in recent years resulted in a substantial increase in open data portals in various fields, compiling the data from different data sources. Some examples of open data portals comprising the ocean domain may be Copernicus or EMODnet. This large collection of heterogeneous data sources is the basis for creating a DTO, as it allows for checking the status of the ocean from a multi-modal perspective at any moment in time.
However, the data provided by these portals is focused on specific data products. Consequently, a tool able to merge multi-modal data is needed in order to provide models taking advantage of the synergies that different data sources may put into relieve when studied together.
DIGI4ECO aims to work with retrospective, present, and prospective data. The real-time data gathered in the underwater observatories to be deployed in the envisioned use cases provide a precise view of the monitored areas’ current status.
In this project, we will develop tools to appropriately merge multi-modal datasets within a unified model in a timely manner that is able to provide useful information for demo sites. Moreover, this correlation between historical and current data will be used to provide a model to foresee the status and relevant changes to occur in the short/long term in the demo sites and nearby areas.
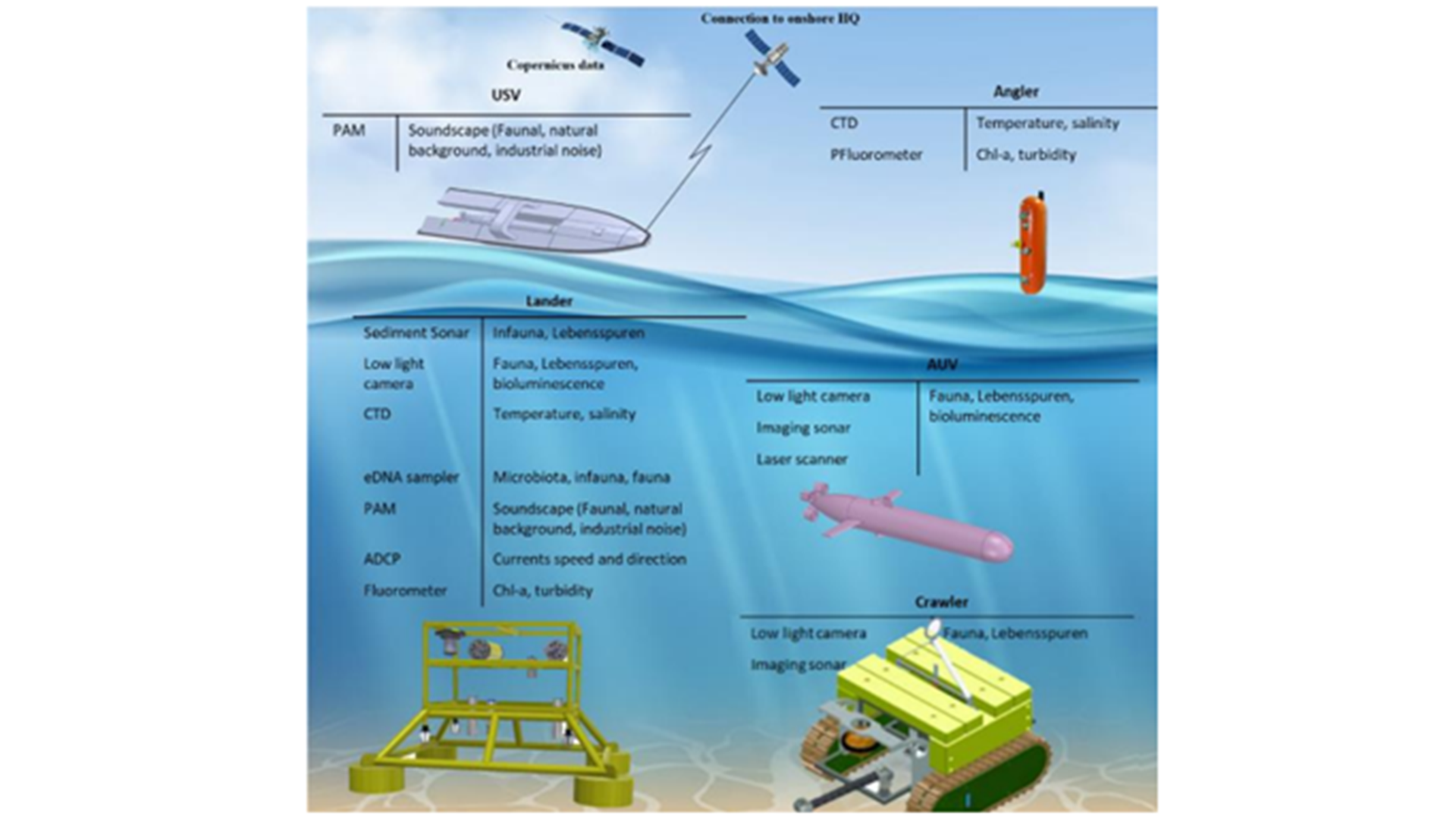
Oceanographic modelling and satellite data through numerical ocean modelling and the Marine Copernicus database, respectively, will be integrated into the DTO to provide analytical and predictive services. A non-exhaustive list of these services includes maps of areas for the demo sites, an analysis of spatiotemporal environmental changes, and oceanographic predictions using near-real-time data as forcing conditions in the models. This will offer predicting capabilities for the DTO, especially after merging historical data with real-time data (demo-mission data).
In data modelling, DIGI4ECO will develop a data ingestion pipeline that can collect all the data from monitoring platforms in the demo sites and operating in nearby areas. The outcome of relating such data with the demo sites’ data will be upscaling a spatial digital twin from discrete information to a useful and regional vision of the marine field conditions.
Other origins of retrospective data from open-source portals such as EMODNet will further broaden the DTO capacity. For instance, the data collected by PTO components will be augmented with retrospective data from EMODnet. DIGI4ECO will also use retrospective optoacoustic data to fill the gaps in data collected by the monitoring platforms. Finally, an open call to data owners, supported by a Cascading Grant system, will be launched for data owners.
Improving non-experts’ accessibility
DIGI4ECO aims to develop socio-ecological models to inform policies and strategies dealing with marine conservation, restoration, planning, and management at multiple spatial scales, harmonising and converting scientific data into manageable information (e.g. jurisdictional instruments) for non-expert users of the DTOs.
Another aspect is removing barriers to the sharing and exploitation of results to extend socio-ecological modelling and make data readily available and more applicable to the public and policymakers. These barriers are psychological and behavioural, as well as the description of information and data and the practical inadequacy of strategies and resources.
Autonomous platforms for 4D monitoring
Currently, ecological monitoring is based on semi-autonomous platforms (e.g. ARGO floats), vessel-assisted robotic technologies (ROVs and AUVs), and deployments of landers. In most cases, their operational autonomy is limited, with no prolonged in-situ data collection capability. This limits the creation of autonomous marine laboratories that would rely on an efficient and sustained coupling of the PT with a DTO as a virtual environment for data management (e.g., collection and elaboration).
DIGI4ECO foresees the implementation of a cost-effective, 4D-autonomous, biological and environmental multidisciplinary data collection capability by a network of robotic platforms (as PT) thanks to the suitable development of a DTO management infrastructure. The project will establish cost-effective monitoring networks of cooperative autonomous fixed and mobile USVs, AUVs, plus benthic crawler robots, and fixed landers platforms, which will operate from the surface, throughout the water column, to the seafloor. DIGI4ECO will expand our spatiotemporal monitoring capabilities, resolution, and reach, providing the suitable DTO infrastructure to manage data flow from robotic technologies equipped with innovative sensor packages: Opto-acoustic imaging, 3D laser-scanning and molecular eco-genomic measurements.
Omics augmented ecological monitoring
New tools for the determination of biodiversity are focusing on eDNA/eRNA detection and semi-quantification. Eco-genomics sensors are being developed in association with microfluidic sampling capability, as well as in-situ molecular laboratories in diverse research fields for the detection of invasive species, waterborne pathogens, and oil and gas tracers.
DIGI4ECO will pave the route for the use of in-situ bio-eco detecting tools and sensors, scouting for the presence of species. Such a development will converge with in-situ microfluidic and time-lapse autonomous sampling capability (presently far from being achieved in the marine environment). Combining imaging and omics approaches will allow the quantification of the presence and activity of organisms within a broad range of ecological sizes.
However, species traceability by omics coupled with concomitant image acquisition requires a careful calibration phase. DIGI4ECO will advance this capability by allowing the detection of a broad range of taxonomic groups based on genetic references, thanks to the integration of AI algorithms that seek equivalents in images.
DTO infrastructure will allow flagging the detection (or absence) of species in both imaging and omics repositories, as well as in comparison to predictive models based on preloaded scenarios (e.g., historical or sleeping repositories).
AI tools for image recognition
Nowadays, deep learning algorithms are the most popular tool for image recognition, and they are a gold standard over classical machine learning methods. Over the last few years, impressive performance improvements have been achieved with Convolutional Neural Networks (CNNs) with respect to any of the traditional classifiers.
Convolutional Neural Networks (CNNs) are a form of Artificial Neural Networks (ANNs) that encode image-specific features into the architecture and are thus particularly adapted to pattern recognition within images (or any other form of 2D data) while having a few parameters required to run the model.
Even though most CNN architectures try to solve classification tasks, it is possible to perform object detection using strategies such as sliding windows, R-CNN or Fast-R-CNN, and You Look Only Once (YOLO). DIGI4ECO will implement new tools for image recognition based on the last improvements in general image detection/classification that have not yet been used in underwater imaging. At the same time, this project will propose a new pipeline that will help scientists label their data and automatically classify them on the cloud (cloud computing approach).
DIGI4ECO has received funding from the European Union’s Horizon Europe programme under grant agreement No 101112883.
Please note, this article will also appear in the 19th edition of our quarterly publication.