Georgia Tech and Meta have collaborated on a massive database to make it easier and faster to implement direct air capture technologies.
Direct air capture has great potential to reduce carbon emissions as it pulls carbon dioxide out of the ambient air.
However, for direct air capture technology, every type of environment requires a specific design.
For example, a direct air capture configuration in Texas would be different from one in Iceland.
These systems must be designed with exact parameters for humidity, temperature, and air flows for each place.
Andrew J Medford, associate professor in the School of Chemical and Biomolecular Engineering (ChBE) and a lead author of the paper, said: “A major problem with direct air capture is finding a material that can capture carbon efficiently under each environment’s specific conditions.”
The new database allowed the team to train an AI model that is orders of magnitude faster than existing chemistry solutions.
The OpenDAC database could accelerate climate solutions the planet needs.
The dataset contains reaction data for 8,400 different materials and is powered by nearly 40 million quantum mechanics calculations. It is one of the largest and most robust datasets of its kind.
The work is published in the journal ACS Central Science.
Building the database through collaboration
Meta’s FAIR team wanted to utilise machine learning to combat climate change, focusing on direct air capture technology.
The team partnered with Georgia Tech to gain expertise in metal-organic frameworks and machine learning applications.
They provided inputs for the database, requiring knowledge of MOF structures and their interactions with carbon dioxide and water molecules.
The development of the inputs used existing MOF structures, including the imperfections found in practical materials.
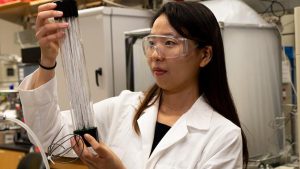
Quantum chemistry and machine learning
The team then generated the database by running quantum chemistry computations on the inputs.
The calculations used around 400 million CPU hours, equivalent to hundreds of times more computing than the average computing lab can do in a year.
Machine learning models were also trained on the database. Once trained on the calculations, the models could predict how the MOFs would react with carbon dioxide.
The team’s AI models were shown to be new tools for material discovery, offering accuracy to traditional calculations while being much faster.
The databases were able to identify around 251 MOFs of exceptionally high potential for direct air capture.
Accelerating direct air capture for the future
Although direct air capture is still a nascent field, the team argues that groundbreaking tools like the OpenDAC database should be in development now.
Anuroop Sriram, research engineering lead at FAIR and first author on the paper, said: “Direct air capture has great potential but needs to be scaled up significantly before we can make a real impact. I think the only way we can get there is by finding better materials.”
Both teams hope that the scientific community will join the search for suitable materials.
The OpenDAC dataset project is open source.