Dominic Couldwell, Head of Field Engineering EMEA at DataStax, explores how businesses can identify opportunities for growth in their generative AI models.
The phrase ‘an old head on young shoulders’ goes back in time, referenced by Dutch scholar Erasmus and in Shakespeare’s Merchant of Venice. It covers how those we consider young and inexperienced can be wise or, at the very least, more concerned about their choices than we might expect. We expect maturity to lead to better results, and we don’t expect new contacts without that maturity and experience to deliver at the same level.
How does this apply to generative AI (GenAI)? One of the main benefits of GenAI is that it can provide more advice and expertise to those who might need it. For example, in the software developer space, we found more than half (55%) of developers already use AI co-pilots to help them code.
In our research report, The State of AI Innovation, we found that for those companies that were ahead in the market around GenAI, the percentage was even higher – 67.5% of developers use AI as part of their work.
Taking the right approach to GenAI
At the same time, GenAI should not be viewed as a threat to how we all work. In our research, 61% thought it would greatly enhance their prospects or create new opportunities for them, while only 1.9% thought that AI was a ‘significant threat’ to the future of their careers. Rather than being a competition between AI and people, the competition is between companies’ employees who make the most of AI and other companies where employees do not.
From developers getting help to solve coding problems to clinical staff getting personalised care responses for patients that they are looking after based on their specific care records, co-pilots should make each interaction a better experience and deliver more effective results. GenAI can help everyone gain that insight and ‘old head on young shoulders’ as part of their work.
However, GenAI itself is still just getting started. For these projects to succeed, there will be other questions to answer. For example, how can you bring your own company data to bear around users or customers? Can you use that data now, and how can you overcome issues around scale, privacy, and trust?
A generative AI maturity model can deliver answers to these questions.
Like any new technology, it can take time to work out how all the moving parts fit together in the most efficient way. This involves looking at more than just the technology itself, and GenAI is no exception.
Indeed, the people and process elements that go alongside Large Language Models will be more important than the LLMs themselves, which are rapidly commoditising as more companies enter the market and offer products. What you can achieve through combining people and technology around better, more automated processes is where more value can be created and captured.
To develop a maturity model for GenAI, you have to track three key areas: Architecture, Culture, and Trust.
The Future AI Stack
One of the foundational elements of success with Gen AI will be the architecture of the AI Stack you build. The common patterns for this are still being established, but key considerations include balancing your real-time and training time data needs, support for multiple different data formats and data privacy. If your infrastructure doesn’t support those features, you’ll be limited in how far you can go.
For example, some generative AI components are stateless – Large Language Models like ChatGPT are rarely updated and remain static. So, if you want to add real-time data to the responses you generate, you must add data streaming elements to your AI Stack. This will provide the LLM with fresh data to include.
The data needs of Gen AI mean bringing structured and unstructured data together, which will involve using different components as part of the overall stack. Unlocking this data allows you to combine foundational models with other approaches to improve your results, such as tuning models based on domain-specific knowledge or calling on other data sets to enhance relevancy and minimise AI hallucinations.
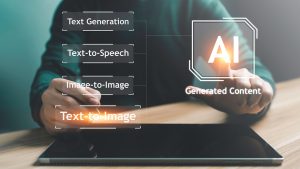
This contextual data has to be managed effectively, from having clean and accurate data in the first place to putting it into the right format to be used with LLMs. Without that preparation, you won’t be able to use Gen AI as effectively as you would like and your responses will be more generic and less satisfying for users. To improve your approach over time, it is important to experiment but also have clear metrics in place that will allow you to measure success and fail fast if required. This should also include a feedback loop so your employees and customers can react to Gen AI-powered services and help improve the service further.
Culture and Gen AI
Alongside the technology element, you also have to consider your approach to people and culture. This involves how you coach the team directly working on your AI initiative and how you improve your processes across the wider organisation. Employees might fear AI and that it might replace them, but they are key to making any technology transformations work in practice.
Implementing generative AI is not an opportunity to replace people with AI. Instead, you should create a competitive advantage by providing your employees with AI support to improve their efficiency and deliver better outcomes. Understanding how Gen AI works and the role you envision for AI Agents as Co-pilots can help your employees understand the challenges you want to solve and encourage them to provide suggestions for improvements. This transparency around how AI is developed and plans to support employees as individuals will determine how they respond as a group.
Trusting in AI
Alongside working on your internal culture around AI, you will also have to consider your external stakeholders, from customers through to regulators and the wider public. Being transparent about how your AI services use data will be critical as AI gets used more widely.
This ongoing effort covers governance, security and data privacy, taking the wider industry landscape into account. Your existing internal data management, privacy and security processes act as the starting point for this work, but you will also have to track new standards and how those will affect your operations.
Public engagement in how those standards are put together provides an opportunity to shape those decisions – this could include working as part of open source communities on the tools that are used to handle data so that they can support data privacy and security, through to lobbying government around regulation and making the argument for safe, secure and effective use of data in AI. Keeping ahead of any changes reduces the cost of being compliant over time.
Maturity and context
A generative AI maturity model can act as a guide for how to develop around this new technology. This approach encourages teams to think about their actions in context, how they can support their teams and people, and how to work around stakeholder and public engagement. Implementing AI can put ‘old heads on young shoulders’ so that everyone can feel more productive and more valuable to the organisation through developing co-pilots for every role.
By thinking bigger in this way, you can foster a culture of experimentation and identify opportunities for future growth. As more companies adopt AI and compete around aiding their employees with AI co-pilots, staying ahead of the curve will be essential to achieve long-term leadership in the market.