Guy Mozolowski, COO of Oxford Cancer Biomarkers, discusses how the leading cancer research facility uses AI to create personalised cancer treatment pathways.
Our mission is to develop precision oncology diagnostic technology, providing patients and clinicians with the tools to create personalised cancer treatment.
We know this will improve patient outcomes and drive cost savings within healthcare systems. Oxford Cancer Biomarkers (OCB) has engineered technology that enables clinicians to better understand a patient’s cancer and tailor their treatment.
Towards personalised cancer treatment
Cancer is a complex, heterogeneous disease driven by multiple, often interrelated factors acting at the genetic, epigenetic, transcriptional, and translated protein levels. The inherent complexity of these factors dictates that different cancers, with varying driving factors, will respond to other treatments and therapies.
Until recently, very few tools have been available to clinicians to interrogate and characterise different factors driving cancer. This has necessitated a largely empirical approach to treatment, i.e. using observation or experience rather than theory or pure logic.
The concept of precision oncology is centred on the principle of patient stratification. In practical terms, this means the ability to characterise clinically significant cancer subtypes and create personalised cancer treatment for the best outcomes. In broad terms, it aims to advance the clinical conversation from ‘How do we best treat cancer?’ towards ‘How do we best treat this individual patient’s cancer?’
OCB designs, builds, and deploys the next generation of precision oncology diagnostic technology. This technology allows clinicians to stratify patent groups that traditionally would have been treated empirically. Therefore, OCB allows its clients to deliver the benefits of precision oncology to their patients.
As pioneers of precision oncology, we use various technologies to develop precision diagnostic technologies, from molecular techniques to cytopathology. Recent developments in machine learning have allowed us to explore ways of interrogating traditional histopathology images to deliver actionable information to the clinic.
This emerging sector, termed ‘digital or computational pathology,’ promises to revolutionise cancer care but brings with it a unique set of technology requirements and challenges.
To address our technology requirements, we must have access to the most appropriate server and storage architecture to run our data and computationally intensive workloads. Platforms deployed for highly performant clinical workloads would benefit from being AI-ready, as healthcare providers are looking at cost efficiencies and improving patient care outcomes.
Digital pathology enables stratification
Digital or computational pathology is the transformation of traditional pathology services using technology. We identified that this field has the potential to positively impact patient care by enabling stratification.
Our work with our technology partner, CSI, aims to enable the stratification of bowel cancer patients. Currently, the majority of mid to late-stage bowel cancer patients (Stage IIb disease and higher) are offered what is known as adjuvant chemotherapy. This is chemotherapy that is given after surgery and has been shown to improve five-year survival rates of bowel cancer patients by up to 8% compared to surgery alone.
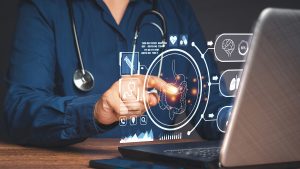
There are, however, disadvantages to this approach; principally, clinicians end up giving a lot of people adjuvant chemotherapy to help a relatively small number. Conversely, it means that a lot of people are given chemotherapy who do not benefit from it but are still at risk of significant side effects.
So, the question was, can we work with CSI to ‘identify and treat the 8% of patients who will benefit from adjuvant chemotherapy and spare those who will not, from unnecessary treatment?’
Under the scope: How the technology works
As part of the normal treatment for bowel cancer, after surgery, a sample of the patient’s tumour is processed in the pathology laboratory of the hospital and examined under a microscope by a pathologist.
In addition to the pathologist’s examination, we can now use our personalised treatment technology to take images of these same tumour samples and subject them to machine learning-based image analysis algorithms. These algorithms identify features in the tumour microenvironment, indicating whether the tumour is aggressive and more likely to recur. Patients whose tumours have these high-risk features are likely to benefit from adjuvant chemotherapy, i.e., they are likely to be among the 8%.
To run the image-based analysis algorithms, we needed a high-performance platform designed for AI deep learning, which CSI provided in the form of an Infrastructure as a Service (IaaS) model based on their PowerCloud. Generally, legacy environments can benefit from the PowerCloud, which becomes an entry point into AI and machine learning. Most environments are purpose-built but may not be futureproofed and ready for the next generation of AI.
Our AI initiatives are also supported by an IBM Power System AC922, combining 40 POWER CPUs with four NVIDIA Tesla GPUs. NVLINK2 technology provides direct CPU-GPU communications, delivering the required high performance. IBM V7000 delivers 14TB of SAN-attached storage for datasets and AI models.
The IBM Power9 server (AC922) also offers Large Model Support where AI models can be trained on images larger than the memory available on the GPU by using system memory – this allows high-definition images to be used. Deep learning model training is compute-heavy, but we can now take advantage of AC922’s distributed training feature, enabling jobs to be broken down and processed in parallel over a cluster of GPUs.
With the right technology in place, we have used the latest AI and machine learning tools to simplify personalised cancer treatment pathways and clinicians’ workflows. This will directly improve patient care by stopping unnecessary treatment and reducing the NHS’s cancer care costs.
We’ve reduced clinical errors and freed up resources so clinicians can spend more time on patient care. We’re proof that scalable, cost-effective digital pathology will drive innovation and improvements in patient care in global healthcare markets.