Jonathan Neal, VP of Solutions Engineering at Saviynt, outlines how intelligence technologies are crucial to preserving identity security.
The recent advent of ChatGPT has created an explosion of interest in Artificial Intelligence (AI) and Machine Learning (ML). While everyone is theorising about the potential use of these technologies, AI and ML already accelerate identity security by streamlining processes and providing actionable insights to administrators and users.
Identity security refers to the measures and techniques used to protect an individual’s or machine’s unique identity and sensitive information from being stolen, misused, or compromised. This type of security focuses on verifying and authenticating the identity of a human or digital user before granting access to certain systems or information. It involves several components, including authentication, authorisation, and access control.
Securing identities is critical in today’s digital age as cyber threats continue to evolve and the risks associated with data breaches and identity theft become increasingly severe. Organisations and individuals must proactively protect their personal identity and sensitive information, including implementing strong authentication mechanisms, regularly monitoring and auditing access controls, and staying up-to-date with the latest security best practices and technologies.
Before looking into how Artificial Intelligence and Machine Learning benefit in bolstering identity security programmes, let’s establish how AI and ML function and the main differentiators of these two technologies.
What’s the difference between AI and ML?
While AI and ML are both fields of computer science that deal with developing intelligent systems, there’s a significant difference between these two technologies.
AI involves creating computer programmes that can perform tasks that typically require human intelligence, such as problem-solving, decision-making, and natural language processing. ML is a subfield of AI that creates algorithms which can learn and improve from data without being explicitly programmed.
The main difference between these two technologies is that AI is a broader concept encompassing different techniques and approaches. At the same time, ML is a specific application of AI that involves training algorithms to recognise patterns in data and make predictions or decisions based on that data.
Next to academic or theoretical AI research, which focuses on developing new algorithms or advancing the field’s fundamental knowledge, applied and generative AI are the two branches that find practical application in day-to-day life, professional or personal.
Applied AI solutions often involve natural language processing, computer vision, or other AI techniques combined with domain-specific expertise and data. This branch is used in various fields, such as healthcare, finance, transportation, manufacturing, etc. ML falls under this branch of AI technology.
Examples of applied AI solutions include fraud detection in financial transactions, predictive maintenance in manufacturing, chatbots for customer service, recommendation systems for e-commerce, and image recognition in healthcare.
Overall, applied AI aims to bring the benefits of AI technologies to practical use cases, improving efficiency, productivity and decision-making in various industries and domains.
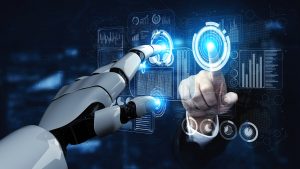
On the other hand, general AI refers to systems that can perform human-like tasks. It is a subset of machine learning that involves training models to generate novel outputs, such as images, videos, music or text.
Using deep learning algorithms to learn patterns and relationships within a dataset, generative AI can create new content similar in style, format, or structure. To work, these algorithms are trained on large datasets, often containing millions of examples, and can produce highly realistic and convincing outputs, as we currently observed with ChatGPT.
Generative AI has potential applications in areas such as healthcare, finance, and autonomous driving, where it can be used to generate synthetic data for testing and training AI models.
Drilling down to identity security, it is ML which can be most readily leveraged to analyse user behaviour, find and mitigate vulnerabilities, and streamline operations.
How ML can be leveraged to bolster enterprises’ identity security programmes
ML technology can provide valuable insights and suggestions based on data analysis, optimising workflows and reducing frustration for administrators tasked with managing identity security programmes.
There are multiple ways in which ML can be effectively applied to this field, for example, by empowering workforces, simplifying management, reducing costs, and more. With its contextual understanding, a system can automatically recommend the next step or revise workflows, leading to improved and streamlined processes, fewer human errors, and stronger overall security.
One instance of how ML benefits identity security is when evaluating access rights and usage patterns. Here, ML enables the system to recommend access throughout an identity’s lifecycle, from the initial request to ongoing micro-certification campaigns.
Furthermore, many of the routine activities related to identity security can be automated, making employee onboarding faster. The system can also offer insights to entitlement owners on how a person’s access compares to that of their peers and other roles, helping expedite approvals and minimise digital exhaustion for administrators and end-users.
Moreover, machine learning can detect unusual behaviour and identity anomalies that may threaten the organisation. By analysing these outliers, access revocations can be automated or used to initiate additional reviews. When developing and maintaining roles, ML can evaluate current roles, identify any similar ones that could be merged, and suggest new roles that may be advantageous.
The further benefits provided by AI
Using analytics, AI and ML to improve enterprise identity security is critical to outpace cybersecurity threats. Rather than buzzwords, leaders want to see real-world use cases where human and machine intelligence meaningfully converge.
AI can bring several benefits to Identity Access Management (IAM), such as:
- Improved security: AI algorithms can enhance security measures by detecting anomalies and suspicious activities in real-time, allowing security teams to respond to potential threats and prevent any potential harm promptly;
- Fraud Detection: AI can detect fraudulent activities related to identity, such as detecting phishing scams, social engineering attacks and fake user accounts. These systems can learn patterns and behaviours associated with fraudulent activities and flag any anomalies;
- Enhanced user experience: By leveraging AI technology, IAM systems can offer a more tailored and intuitive experience by adapting to the user’s behaviour, preferences and requirements; and
- Cost reduction: AI can assist organisations in reducing access management and security incident response costs by automating repetitive tasks and minimising the requirement for human intervention.
AI and ML can revolutionise security systems
AI and ML have the potential to revolutionise identity security and speed up the adoption of related programmes by providing actionable insights and streamlining processes.
Identity security is critical in today’s digital age, where cyber threats continue to evolve, and the risks associated with data breaches and identity theft become increasingly severe.
ML can automate routine activities related to identity security, detect unusual behaviour and identity anomalies, evaluate access rights and usage patterns, and offer insights to entitlement owners.
Additionally, AI algorithms can enhance security measures and enhance user experience by reducing the time and effort required to manage IAM programmes. Utilising these capabilities, organisations can quickly identify and address high-risk access and activities, ensuring regulatory compliance on an ongoing basis.
Integrating AI and ML in identity security programmes can improve efficiency, productivity, and decision-making, enabling organisations and individuals to protect their personal identity and sensitive information. Moreover, organisations can shrink their threat landscape by reducing over-privileging and human error.
Jonathan Neal
VP, Solutions Engineering
Saviynt