University of Notre Dame researchers are using AI and Google Street View to predict home energy prices.
Collaborating with experts from the universities of Maryland and Utah, the researchers are utilising AI techniques to analyse a household’s passive design characteristics to calculate home energy prices.
The team believes the research will help inform policymakers and urban planners in identifying vulnerable communities, creating more sustainable building designs, and reducing the growing energy burden on citizens.
Siavash Ghorbany, a doctoral student in the Department of Civil and Environmental Engineering and Earth Science, explained: “The first step toward mitigating the energy burden for low-income families is to get a better understanding of the issue and to be able to measure and predict it.
“So, we asked, ‘What if we could use everyday tools and technologies like Google Street View, combined with the power of machine learning, to gather this information?’ We hope it will be a positive step toward energy justice in the United States.”
The growing energy burden for US households
Low-income households across the US are grappling with an energy burden that is significantly higher than the national average, recent findings from the US Department of Energy reveal.
A report found that more than 46 million households in the US are experiencing a substantial energy burden, defined as allocating over 6% of their gross income towards basic energy needs such as heating and cooling their homes.
How passive design can reduce home energy prices
Passive design strategies, such as natural ventilation and optimal use of sunlight, have emerged as potential solutions to mitigate home energy prices.
However, the scarcity of data on passive design effectiveness poses a challenge in assessing its impact on a broader scale.
To address this knowledge gap, a team of interdisciplinary experts devised a novel method employing AI.
Scalable model for energy auditing
The research concentrated on three pivotal elements in passive design—window size, window type (operable or fixed), and the extent of shading. The study utilised a convolutional neural network to examine Google Street View images of residential structures in Chicago.
Subsequently, various machine learning techniques were employed to determine the most effective prediction model. Their approach predicted home energy prices with an impressive accuracy rate exceeding 74%.
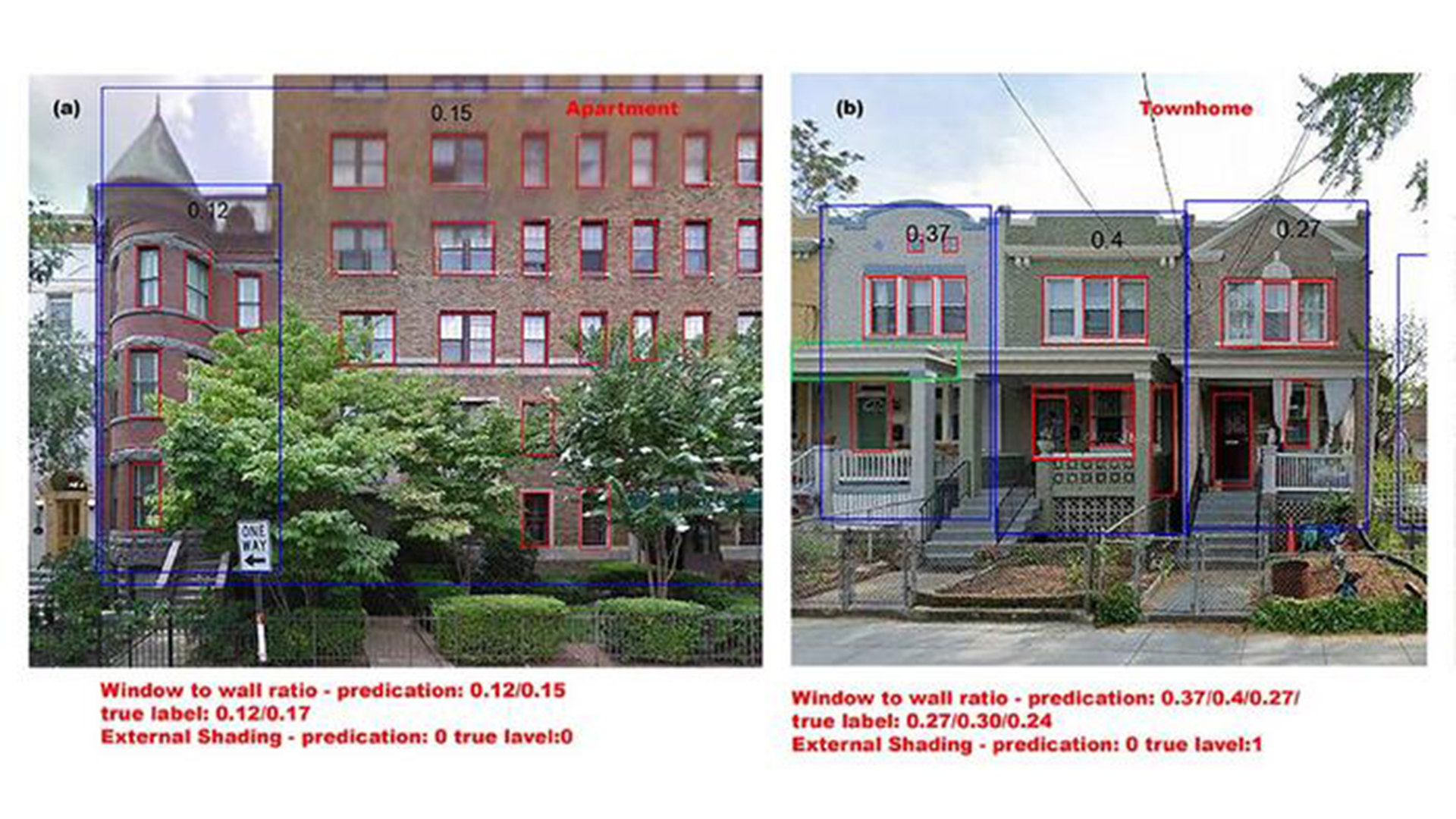
Unlike conventional approaches that require meticulous building-by-building assessments, this model enables swift and scalable evaluations.
The findings underscore the significance of passive design attributes in predicting average energy burden, emphasising their indispensable role in predictive modelling.
Moving forward, the researchers aim to expand their analysis to include additional passive design elements such as insulation and green roofs.
Moreover, they envision scaling up the project to address energy burden disparities at a national level, with the ultimate goal of fostering sustainable and equitable energy practices across the country.