Adam Lieberman, Head of Artificial Intelligence and Machine Learning at Finastra, outlines how explainable AI can help remove bias – important in the age of ChatGPT.
From the inception of Artificial Intelligence, the technology has been the source of intermittent excitement, worry, and, of course, advancement across industries.
From Skynet to revolutionary diagnostics capabilities in healthcare, AI has the power to both capture the imagination and drive innovation.
For the general public, discussions around AI usually centre on outlandish doomsday scenarios, concerns about robots taking our jobs, or excitement at how automation may precipitate a more balanced work-life paradigm. For most, the practical application and understanding of AI has largely been hidden from sight, which has led to misapprehension filling the vacuum.
The most compelling use cases for AI have long been the preserve of businesses, governments, and technology giants, but this all changed with the arrival of OpenAI’s ChatGPT. This is the first example of a large language model and its generative capabilities being widely available for mass consumption.
It has created an AI playground that is immediately, and to varying degrees, useful in many contexts.
The most glaring issue, however, and one that has been around since the dawn of AI, is bias.
In recent times, data scientists have put their shoulders to the wheel as they look for ways in which bias can be removed from models, with particular pressure in industries where the outcomes of models might adversely affect customers and end users.
When it comes to financial services, for example, decision-making algorithms have been used for many years to expedite decisions and improve services. But in the context of loans, ‘bad’ or ‘wrong’ decisions that are the product of a biased model can have disastrous consequences for individuals.
Eliminating bias requires a multi-pronged strategy, from ensuring data science and Machine Learning teams are representative of the communities they are building solutions for—or at the very least understand the principles of building fairness into models—to ensuring models are explainable.
The key motivation behind explainable AI as a best practice is the elimination of ‘black box’ Machine Learning models. Black boxes might often be high-performing, but if their outcomes cannot be understood, there can be little concrete defence against charges of inaccuracy or discrimination.
In industries in which decision-making models can have profound consequences, the pressure for increased accountability is growing from both consumers and regulators, which is why, in my view, businesses must seek to get ahead of the curve.
Tips for explaining AI and removing bias
The key components of a model that need explaining when considering bias are often neglected. Data scientists and Machine Learning engineers have a standard pipeline to work to when building a model. Data is, of course, at the heart of everything, so we start by exploring our data sets and identifying relationships between them.
We then go through exploratory data analysis that allows us to turn data into a usable form. Then it’s time to wrangle, clean, and pre-process the data before we begin feature generation to create more useful descriptions of the data to solve the problem at hand.
We then experiment with different models, tune parameters and hyperparameters, validate the models, and repeat the cycle until we have a high-performing solution. The problem here is that without a committed effort to ensure fairness at each stage, the resulting outcomes may be biased.
Of course, we can never ensure the full removal of bias, but we can make efforts to ensure each stage in a model’s development conforms to a methodology that prioritises fairness.
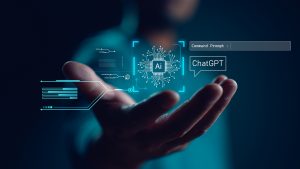
My recommendation for delivering this is to firstly select diverse data sets for training models, i.e. those that are most representative, and also to develop standardised processes and documentation that explains models and how they conform to the methodology, so that the performance and decisions can be understood.
The real challenge here, and a core principle behind explainable AI, is that the inner workings of models should not just be understood by data scientists. In most contexts, multiple parties will need to know (and should know) how a machine learning model works.
Google pioneered the approach to creating standardized documentation that does just this by releasing its paper on ‘Model Cards’ in 2019. In the paper, the authors suggest logging model details, intended use, metrics, evaluation data, ethical considerations, recommendations, and more.
Using this as a basis, and taking into account unique requirements for industries, such as those that are heavily regulated, organisations can show how bias has been systematically accounted for at each stage of a model’s construction. If we return to the use case of a loan provider, it becomes clear why explainable AI is so important.
If a person feels that they have been unfairly denied a loan, it’s important that the loan provider is able to explain why the decision was made. In extreme cases, failure to justify the decision could result in legal action on the grounds of discrimination.
In this instance, it’s important that the model, the methodology used to construct it, and its output, can be understood by legal professionals, as well as the individual affected. Outside of this exceptional case, the information about models may be pertinent to a number of business units and non-technical personas, so different documentation should be tailored to each.
The future of explainable AI
Ultimately, if documentation that explains models does not exist, every model will be a black box solution to someone, which is an increasingly untenable situation. It’s no mistake that ChatGPT has arrived at a time in which the general population has reached a reasonable understanding of data protection, management, and rights. The two may not be directly linked, but can rather be seen as complementary countervailing forces in the evolution of technology.
Thanks to data regulations, such as the EU’s GDPR and California’s Consumer Privacy Act, and the interminable cookie permissions forms on websites, we are all more aware of the data we are sharing and how it might be used—and with greater awareness comes greater expectations.
ChatGPT has fired up the collective imagination of what’s possible when a model is trained on vast amounts of data, but there have been very stark examples of how the model has delivered problematic results. ChatGPT is a black box, so the results it delivers cannot be fully explained or relied upon. It also makes factual errors, some of which are serious, because it generalises from common patterns used in conversation, such as when individuals confidently assert opinion as fact.
As AI continues to evolve, so too will the general understanding of its power and limitations. Large language models are inherently black boxes, which means the future of the likes of ChatGPT, and its usability, will rely on the creation of robust methodologies for inferring how and why these models arrived at their outputs, which is the next stage in explainable AI.