By analysing onboard vehicle data using explainable Artificial Intelligence, Professor Will Northrop from the University of Minnesota envisions a better electric transportation system.
The accelerating global transition to electric transportation is motivated by the need to decarbonise the industry while creating new economic opportunities and reducing adverse human health impacts of the current fossil fuel paradigm. Currently, more than 1.4 billion vehicles are estimated to be in use globally, but less than 1% of them are powered solely by electricity. Electrifying the entire road transportation sector, from e-bikes to heavy duty trucks, is an immense challenge that requires technology breakthroughs for both electric vehicles (EVs) and the infrastructure they rely on.
As battery costs continue to decrease and more cost-effective EVs are introduced, research must focus on equitable adaptation – including households without reliable at-home charging and small businesses with limited resources to support commercial EVs. Planning software, like Argonne National Laboratory’s Electric Vehicle Charging Justice40 mapping tool, seeks to identify locations for additional investment in charging infrastructure to ensure fair EV access. Analysis is also required to ensure that increasing the number of EVs on the road does not further harm those in disadvantaged communities through increased emissions of greenhouse gases and conventional pollutants from additional fossil electricity production.
Onboard vehicle data (OVD), including global positioning system location, powertrain parameters, and other associated data have been used to optimise individual vehicle performance. Although vehicle manufacturers and fleet operators collect large quantities of OVD from modern vehicles, it largely goes underutilised. In recent years, Artificial Intelligence (AI) models trained using OVD have been employed by manufacturers to estimate when a vehicle will need to be charged. However, these models can be improved by making them more explainable. This is done through incorporating estimation of important physical vehicle parameters, like vehicle mass – a critical feature in heavy-duty commercial vehicles. Vehicle data can also be used to understand where infrastructure should be placed for maximum benefit to all drivers, and to estimate emissions hotspots from electricity generation needed for EV charging.
The need for explainable AI in transportation systems
In the past decade, Machine Learning models have become ubiquitous in a variety of fields. Among the models, artificial neural networks (ANNs) are popular due to their ability to approximate any function, if given enough training data and computational power. While such ‘black-box’ AI models are convenient for solving transportation problems, they also present disadvantages. For example, a multi-layered feed-forward ANN, trained on experimental data, can produce strong correlations. However, it is difficult to reason out the fundamental cause for a predicted output. In other words, it is difficult to map a matrix of numbers which constitutes the tunable parameters of the model to an explainable physical phenomenon that is causing the model to output a certain value. This leads to a trade-off between the model’s performance and its transparency, which is the focus of study in the field of explainable AI (XAI).1 The U.S. Defense Advanced Research Projects Agency (DARPA) has stated: “There seems to be an inherent tension between Machine Learning performance (e.g. predictive accuracy) and explainability; often the highest-performing methods (e.g., deep learning) are the least explainable, and the most explainable (e.g. decision trees) are less accurate.”2
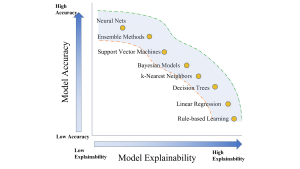
Machine Learning models are critical in transportation applications where high-impact decisions need to be made from the results of these algorithms. For example, a small variance in an algorithmic prediction that results in a semantic misclassification in the vision system of an autonomous car could lead to extreme and dangerous consequences.3 Risk of failure results in accountability and liability concerns. Another important factor is customer trust. Knowledge that algorithm decisions running on an intelligent machine are inherently unexplainable compounds its untrustworthiness. As an example, integration of virtual agents and explainable AI in speech recognition has been shown in research to increase trust in intelligent autonomous systems.4
Explainable AI is essential for transportation engineers during product development and planning. Along with the predictive capabilities of black-box AI models, engineers also require knowledge of the underlying physical phenomenon behind the predictions. This knowledge is key to understanding the system understudy which can further be deemed valuable in design. For example, when attempting to accurately predict nitrogen oxide (NOx) emissions from a conventional internal combustion engine (ICE) vehicle, understanding of operating parameters that cause predicted NOx levels to increase can also be valuable.5 Such models can also be used effectively to predict battery state of health as a function of driver behaviour and ambient conditions in EVs.
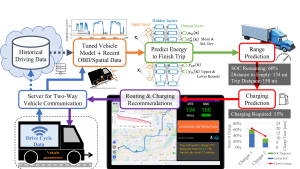
Solving range anxiety across vehicle classes using intelligent energy management
Powertrains in EVs are simpler than their conventional combustion-driven counterparts, but their batteries raise new challenges. Current EV batteries are not energy-dense enough to provide the same driving range as liquid hydrocarbon fuels, especially for large commercial vehicles. Battery life and performance varies significantly depending on ambient storage and operating conditions. Further, battery and cabin thermal management systems needed to mitigate extreme temperatures draw their energy from the traction battery, further impacting EV range. Situationally dependent vehicle range is relatively foreign to new EV owners, and inaccurate range estimates can negatively impact their decision making and overall experience. This has driven research efforts to improve EV range prediction using explainable AI methods that can account for temperature-related fluctuations in auxiliary energy use and battery performance.6
Energy management in EVs includes both accurately predicting driving range and optimising when and where to charge. Because EVs take a relatively long time to recharge compared to refuelling, and fast charging stations are still scarce in many areas, EV owners need guidance to help them determine the best place and time to charge. Trip routing algorithms for EVs should consider charging as an inherent component of their utility. Intelligent charging decisions rely on accurate estimates of EV range, electricity costs, and charge times at available charging stations. To address these problems, Machine Learning models have been created to predict range and charging requirements (i.e. to complete a given route) of an EV using collected OVD as training data.7 Such models may then be used to inform energy-efficient routing decisions, incorporate smart opportunity charging as needed, and provide drivers with accurate time and cost estimates for each trip.
Enhanced access to electric trucks for small regional fleets through data democratisation and optimisation tools
Range challenges facing light-duty EVs are exacerbated in larger commercial vehicles – larger batteries increase vehicle weight and lengthen charge times, and heavier payloads reduce driving range. Furthermore, very few existing charging stations can support both power requirements of larger commercial EVs and adequate parking space.
While significant investments in commercial EVs have been made by large companies, smaller firms cannot afford to spend limited resources on uncertain investments like converting to EVs. Even determining whether a truck fleet can be electrified is challenging for smaller businesses like grocery distributors or freight delivery services. Publicly available tools and datasets are needed to allow small commercial fleet operators to understand whether electric transportation is functionally and financially viable. Democratisation of these tools and the vehicle data used in them will help determine the feasibility of electrifying vehicles in small truck fleets and quantify the benefits and costs to businesses and the environment. To be effective for medium- and heavy-duty fleets, critical parameters like payload mass must be considered in energy-related calculations and accompanying charger sizing and placement recommendations should weigh the operational cost of time delays due to charging with upfront costs of installing high-powered chargers.
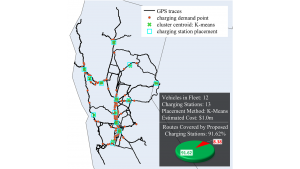
Many commercial fleets currently collect OVD from their conventionally powered fleets to aid in logistical planning and data analysis. Efforts by the U.S. National Renewable Energy Laboratory and others to collect, store, and freely distribute OVD from commercial fleets is critical to aid research efforts in this area.8 Some preliminary commercial9 and private10 tools exist that seek to determine whether an EV powertrain would best fit a given vehicle or route, given some context from historical fleet operations. The newly developed Heavy-Duty Electric Vehicle Integration and Implementation (HEVII) tool aims to provide an open-source option for fleet managers and researchers to determine the electrification potential of vehicles within fleets and the infrastructure required to support future EVs. The HEVII tool incorporates real-time mass estimation, charger placement optimisation techniques, along with financial and emissions analyses. Future work may broaden the scope of such tools beyond fleet-level electrification strategies to consider other parts of the transportation sector (e.g. consumer and public transportation vehicles) and the full vehicle lifecycle (e.g. materials production, vehicle manufacturing, and recycling).
Reducing unintended impacts of increased electric vehicle charging on disadvantaged communities
Collected OVD can be used to inform important environmental justice concerns raised in the transition to electric transportation, comparable to how it has been used for predictive and diagnostic purposes in conventional engine powered vehicles. NOx emissions from transit buses and other vocational vehicles can be tracked spatially to understand transportation emissions exposure hotspots in disadvantaged communities.11
Similarly, OVD can be used to trace unintended consequences of EV usage in the same geographic areas.
While public EV charging stations are becoming more commonplace, their distribution – like that of EVs themselves – has been vastly uneven and skewed towards wealthier, highly-populated areas, and areas with strong government initiatives, leaving many consumers without suitable charging options. Many methods have been proposed to help optimise the placement of new EV charging stations, though there is no standard definition for ‘optimal’ in this context.12 Many methods consider how chargers can be placed to support as many EVs or routes as possible, but few consider the equitability of such charger placements. OVD collected by manufacturers and resold by third parties like Wejo can be used to track charging patterns in households without access to at-home chargers to inform more equitable distribution of inexpensive charging stations.
Beyond determining equitable access to charging in disadvantaged communities, one should consider power generation infrastructure, the potential impact of equitable charger placement on EV adoption, and the subsequent impact of electric transportation on community-level emissions of harmful pollutants like nitrogen oxides and particulate matter from electricity generating facilities. For example, some US states have a high proportion of historically disadvantaged communities, a combustion-heavy energy mix, and very little public charging infrastructure. If EV adoption increases rapidly in these states, areas with more EVs should see an improvement in air quality from reduced tailpipe emissions, but areas near combustion-based power plants might see a simultaneous increase in emissions as energy production increases to meet growing electricity demand. Considerable research effort is needed to investigate how government intervention, the placement of charging infrastructure, and investment in renewable power generation can lead to a fairer and more just transition to electric transportation.
References
- Dosilovic, F K, Brcic, M, & Hlupic, N (2018). Explainable artificial intelligence: A survey. 2018 41st International Convention on Information and Communication Technology, Electronics and Microelectronics (MIPRO), 0210–0215 https://doi.org/10.23919/MIPRO.2018.8400040
- Gunning, D & Aha, D (2019). DARPA’s explainable artificial intelligence (Xai) program. AI Magazine, 40(2), 44–58 https://doi.org/10.1609/aimag.v40i2.2850
- Hussain, F, Hussain, R, & Hossain, E (2021). Explainable artificial intelligence (Xai): An engineering perspective. arXiv. https://doi.org/10.48550/arXiv.2101.03613
- Weitz, K, Schiller, D, Schlagowski, R, Huber, T, & André, E (2019) “Do you trust me?”: Increasing user-trust by integrating virtual agents in explainable ai interaction design. Proceedings of the 19th ACM International Conference on Intelligent Virtual Agents, 7–9 https://doi.org/10.1145/3308532.3329441
- Panneer Selvam, H, Shekhar, S, & Northrop, W F (2021). Prediction of no x emissions from compression ignition engines using ensemble learning-based models with physical interpretability. SAE Technical Papers. 2021-24–0082 https://doi.org/10.4271/2021-24-0082
- Eagon, M, Trujillo, J, & Northrop, W (2022). Modeling climate control loads and the impact on vehicle range for last-mile electric delivery trucks in cold climates. Technical Papers. Paper presented at SAE World Congress (WCX) 2022, Detroit, MI https://doi.org/10.4271/2022-01-0199
- Eagon, M J, Kindem, D K, Panneer Selvam, H, & Northrop, W F (2022). Neural network-based electric vehicle range prediction for smart charging optimization. Journal of Dynamic Systems, Measurement, and Control, 144(1), 011110 https://doi.org/10.1115/1.4053306
- “Fleet DNA Project Data.” (2022). National Renewable Energy Laboratory. Accessed September, 2022: www.nrel.gov/fleetdna
- “ezEV Analytics.” (2022). Sawath Labs. Accessed September, 2022: https://marketplace.geotab.com/solutions/sawatch/
- Moawad, A, Li, Z, Pancorbo, I, Gurumurthy, K M, Freyermuth, V, Islam, E, Vijayagopal, R, Stinson, M, & Rousseau, A (2021). A real-time energy and cost efficient vehicle route assignment neural recommender system. https://doi.org/10.48550/ARXIV.2110.10887
- Kotz, A J, Kittelson, D B, & Northrop, W F (2016). Lagrangian hotspots of in-use no x emissions from transit buses. Environmental Science & Technology, 50(11), 5750–5756 https://doi.org/10.1021/acs.est.6b00550
- Eagon, M, Fakhimi, S, Lyu, G, Yang, A, Lin, B, & Northrop, W F (2022). Model-based framework to optimize charger station deployment for battery electric vehicles. 2022 IEEE Intelligent Vehicles Symposium (IV), 1639–1648 https://doi.org/10.1109/IV51971.2022.9827442
- Zhou, Y, Gohlke, D, Sansone, M, Kuiper, J, & Smith, M (2022). Using mapping tools to prioritize electric vehicle charger benefits to underserved communities (ANL/ESD-22/10, 1870157, 175535). Argonne National Laboratory. https://doi.org/10.2172/1870157
Please note, this article will also appear in the twelfth edition of our quarterly publication.